Xiaoman Zhang1,2
|
Weidi Xie1,2
|
Chaoqin Huang1
|
Ya Zhang1,2
|
Xin Chen3
|
1CMIC, Shanghai Jiao Tong University
|
2Shanghai AI Laboratory
|
3Huawei Cloud
|
Abstract
This paper targets on self-supervised tumor segmentation.
We make the following contributions:
(i) we take inspiration from the observation that tumors are often characterised independently of their contexts, we propose a novel proxy task ``layer-decomposition", that closely matches the goal of the downstream task, and design a scalable pipeline for generating synthetic tumor data for pre-training;
(ii) we propose a two-stage Sim2Real training regime for unsupervised tumor segmentation, where we first pre-train a model with simulated tumors, and then adopt a self-training strategy for downstream data adaptation;
(iii) when evaluating on different tumor segmentation benchmarks,e.g., BraTS2018 for brain tumor segmentation and LiTS2017 for liver tumor segmentation, our approach achieves state-of-the-art segmentation performance under the unsupervised setting. While transferring the model for tumor segmentation under a low-annotation regime, the proposed approach also outperforms all existing self-supervised approaches;
(iv) we conduct extensive ablation studies to analyse the critical components in data simulation, and validate the necessity of different proxy tasks. We demonstrate that, with sufficient texture randomization in simulation, model trained on synthetic data can effortlessly generalise to datasets with real tumors.
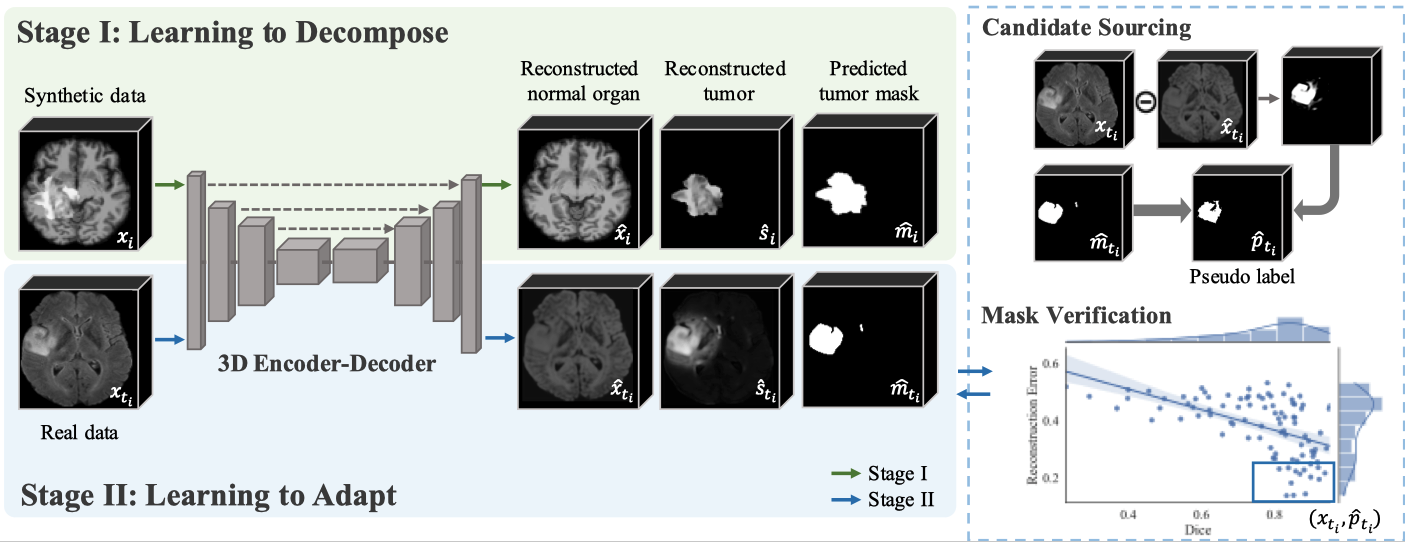
Data Synthesis
Illustration of the data simulation pipeline.
(a) Data simulation pipeline. We first simulate the tumor with a transformed image and a generated 3D mask, which provide texture and shape respectively. Then the synthetic data is composed by blending the simulated tumor into the normal image.
(b) Visualisation of shape simulation. The first row presents the 3D polyhedrons with various shapes, sizes and locations, and the second row shows the binary masks.
(c) Visualisation of texture simulation. We have displayed a randomly selected normal image undergoing different transformations functions (top row), the corresponding simulated tumor (second row), and synthetic training data (bottom row) in columns 2-8.
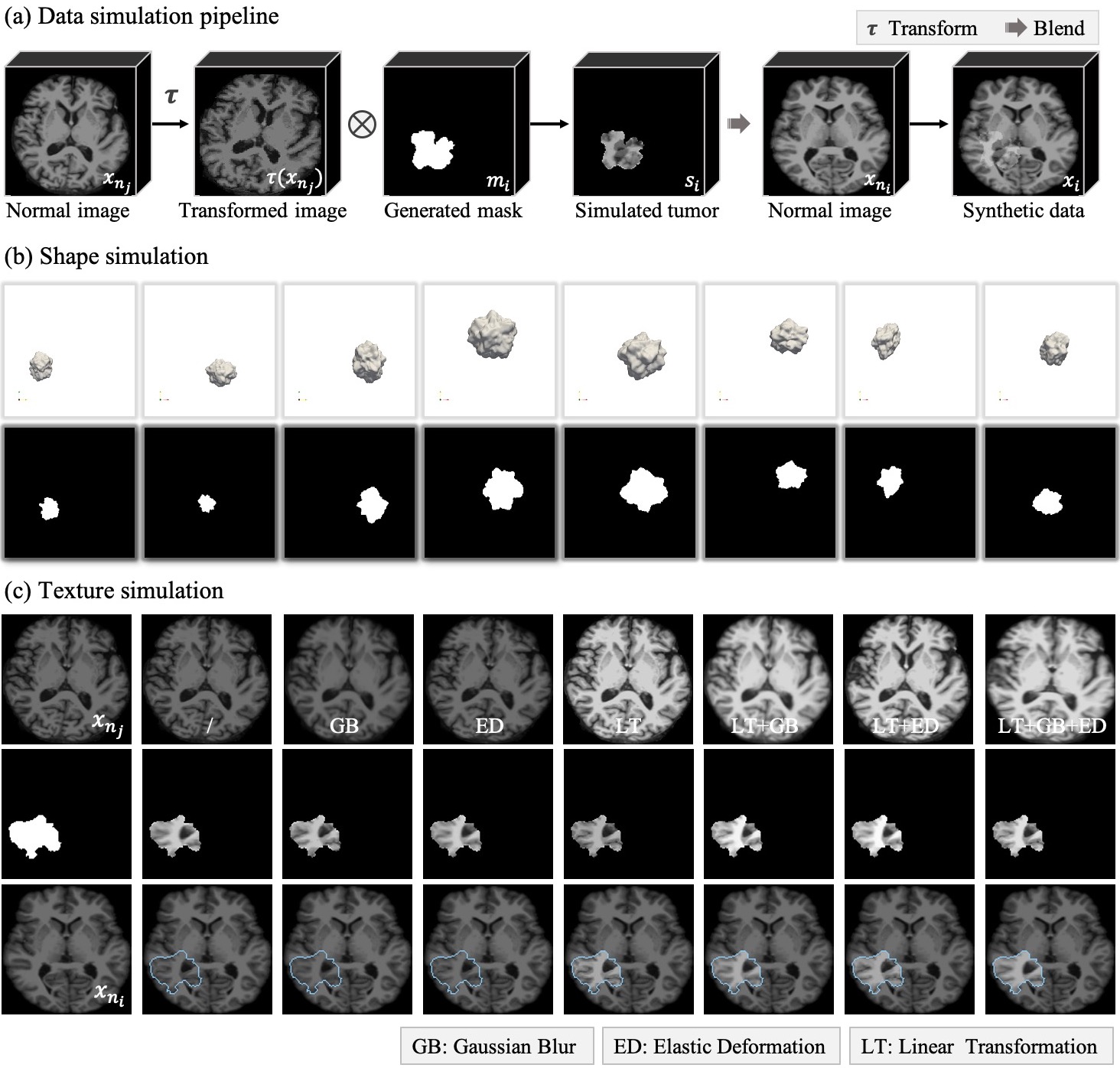
Visualizations of Zero-shot Tumor Segmentation
2D Visualization
From left to right: input volume, ground truth (green) vs. predicted mask (red), reconstructed normal organ, reconstructed tumor, predicted mask.
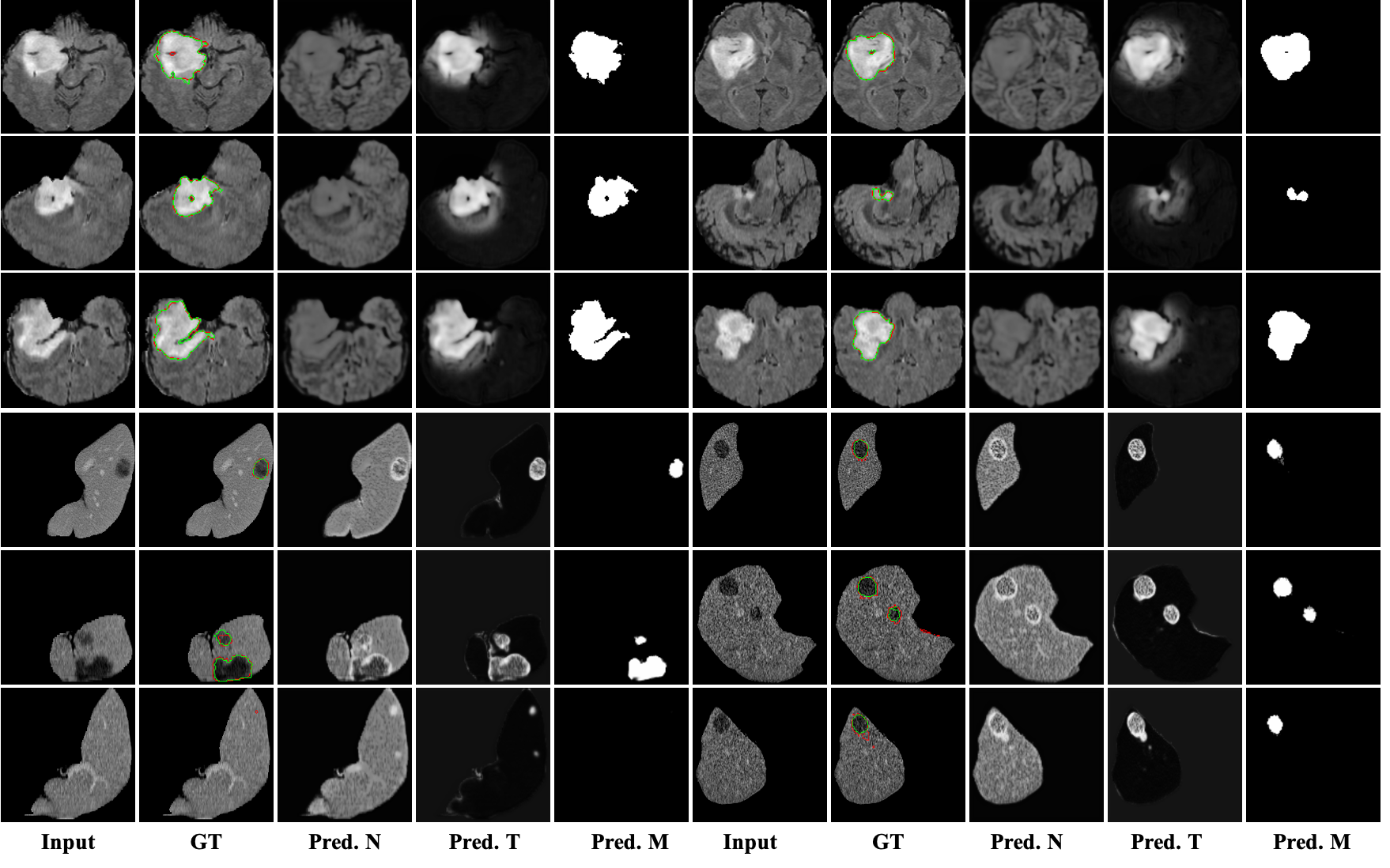
3D Visualization
From left to right: input volume, ground truth, reconstructed normal organ, reconstructed tumor, predicted mask.
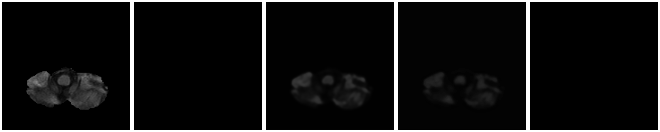
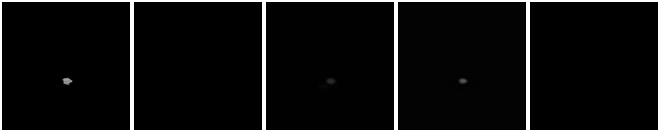


Results
R1: Fully Supervised Fine-tuning
Compare with SOTA self-supervised methods on WTS (brain whole tumor segmentation) and LTS (liver tumor segmentation).
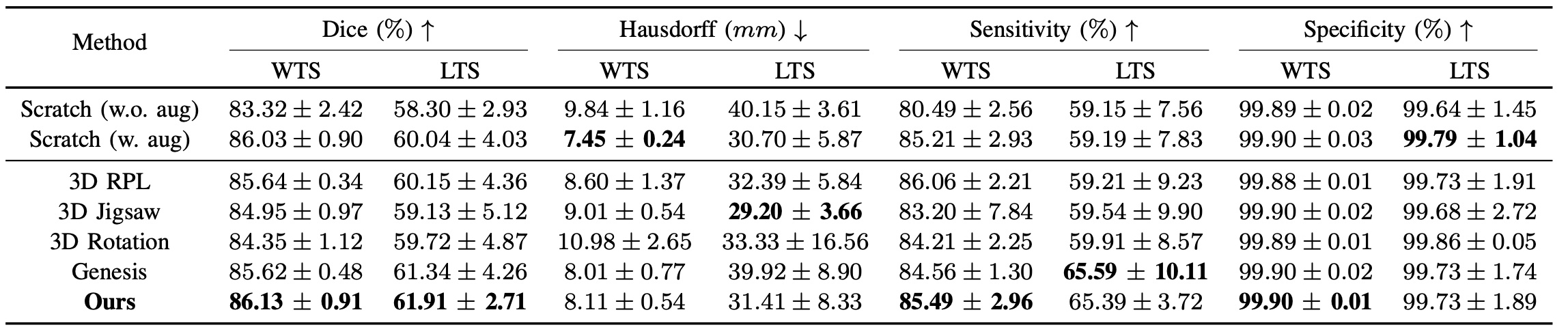
Compare with SOTA self-supervised methods on BTS (Whole tumor, Tumor core and Enhanced tumor segmentation).
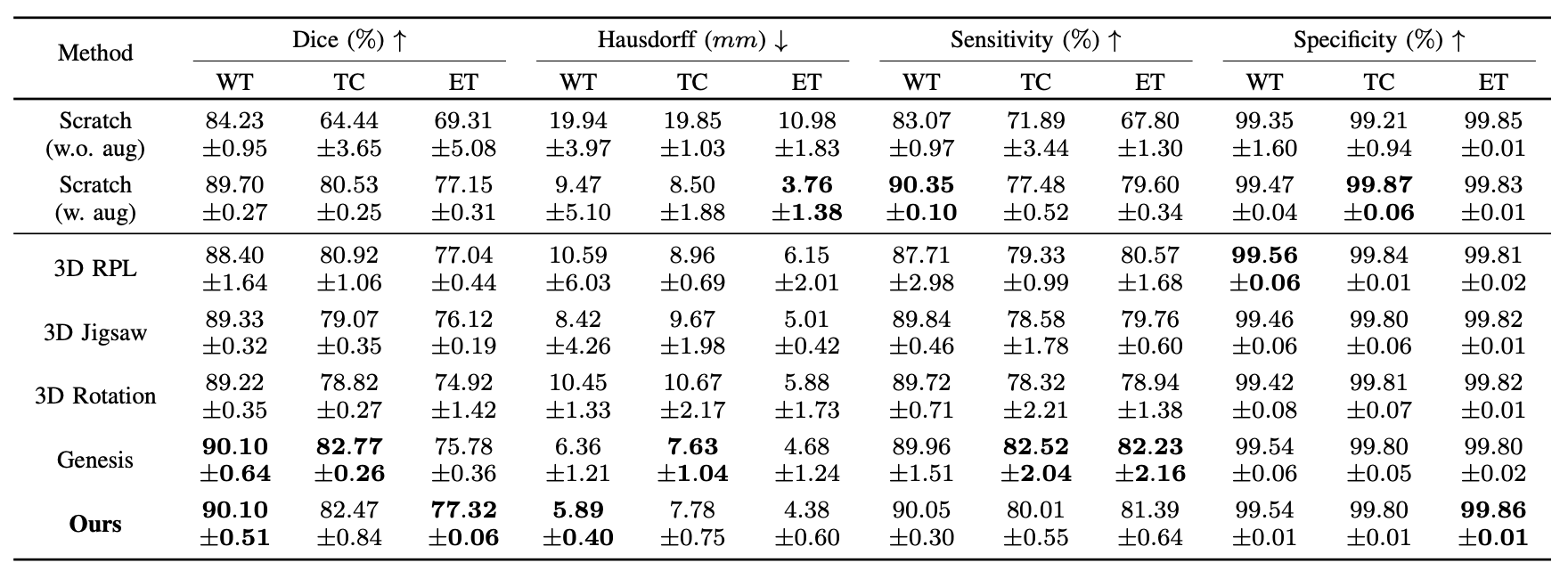
R2: Analysis of Model Transferability
We study the usefulness of self-supervised learning by varying the number of available volume annotations.
For both tasks, our proposed method shows superior performance on all supervision level.

R3: Results of Zero-shot Tumor Segmentation
We compare with approaches that advocate zero-shot tumor segmentation, i.e. the state-of-the-art unsupervised anomaly segmentation methods.
As shown in the Table, our approach surpasses the other ones by a large margin.
Acknowledgements
Based on a template by Phillip Isola and Richard Zhang.